The world of analytics has taken a lot of turns over the past several years. It went through a name change among analytics professionals - the Web Analytic Association changed its name to Digital Analytics Association years ago to better reflect the wider application of website analytics solutions from Google, Adobe, and others.
Today machine learning techniques are injected life into predictive analytics and customer analytics, both becoming one and the same in many instances.
Predictive analytics has existed for decades, but it has received increased attention as cloud computing expanded capability and access to that capability. Open source programming added collaboration among professionals, injecting developer technique and solutions. This has marshaled talents and resources and that has ushered in machine learning techniques in almost every industry and research.
With these factors in mind, analytics is experiencing advances in capability and opportunities associated with that capability. Let’s note what has been emerging so far:
-
Data Mining Is Now A Critical Early Step - The need to review that data has always been important since the day Google bought Urchin and when Adobe Analytics was Omniture Site Catalyst. But that need has been altered as smart devices from phones to cars have become networked to the internet. Most analysts using Google Analytics or Adobe Analytics were concerned with interpreting where website traffic arrived. Now the data sources have shifted to include devices, producing more unstructured data. This increases the need for cleansing techniques to sort data before it is used in machine learning models, which thrive on vetting test data to build model accuracy.
-
The growth of tools in the marketplace that can easily blend data. Statisticians have long used scripting programming languages to build predictive models. Over time vendors have been making inroads in making the interface to those languages easier to visualize results and highlight data errors that can impact model accuracy. Graph databases such as Neo4j are an example of that convenience. Neo4j presents data relationships in a node diagram, easing the highlight of potentially correlated data. Software providers from all levels of technology have introduced tools to fit every skill set and need. Most solutions offer enhanced dashboard visualization and can merge data from several tools and databases. These features are useful for establishing advanced analytic techniques. The best way to consider these tools is to ask which tools best visualize and update the data conveniently.
-
Tech innovators are not exclusively producers of computer or people-facing software - Remember the days when developers debated about the merits of PC versus Mac? Such technological Berlin Walls have fallen long ago, leading to surprise and delight moments. For example, I remember being surprised at the scope of the Capital One appearance at OSCON 2016. Their purpose? To attract developers to an AWS-based solution they created called Cloud Custodian. Today businesses that are adopting open source recognize the need for fostering collaboration among developers. That will lead to new solutions coming from unsuspecting sources.
-
Recommendation engines are refining analytics solution: A/B testing is meant to highlight when one web element is preferred over another. But accuracy in an A/B test can be difficult to achieve when the test is conducted manually. Sometimes users end a test with only a few samples of data when more would have provided more statistically correct accuracy. To raise accuracy in testing, analytic solution providers have introduced machine learning algorithms in serving the test elements for a website, app, or paid media campaigns. This ensure that various layouts, images, and texts define not only presented offers, but also impact the larger customer experience. Since machine learning algorithms generate responses, the payoff for optimization testing is better accuracy from test results. Current solutions are starting to come to market with this capability. Adobe announced an Auto-Target, an A/B testing feature that uses a machine learning protocol to automate personalization testing of elements and learn the preferred individual experience with media.
-
The rise of deeper statistics. Much of the tests available for websites and app optimization centered on frequency-related stats for tasks such as establishing sample size for an A/B test. The advancement of cloud computing, coupled with data visualization options, has allowed for better application of conditional statistics. Condition statistics look at the likelihood of an activity given the existence of a certain condition. The “condition” facing marketers can incorporate factors such as demographics or location, thanks to the rise of mobile and IoT devices. This is an important distinction from frequency-based statistics, which does not account for a condition. Cloud computing has also paved a way for once-arcane statistics theories, like the One-Armed Bandit and Simpson’s Paradox to be more applicable to data mining, data segmentation, and ultimately real-life challenges that are more data-based. Programming languages such as R and Python can display these theories and their application in action.
Despite these advances, one asterisk remains: Marketers and analysts still require the skills and judgment to make sure the software reports accurate data. With companies expected to spend on analytics in general over the next 3 years, you should expect skills to increase appropriately. Marketers should participate in work conferences for tool as well as find ways to collaborate.
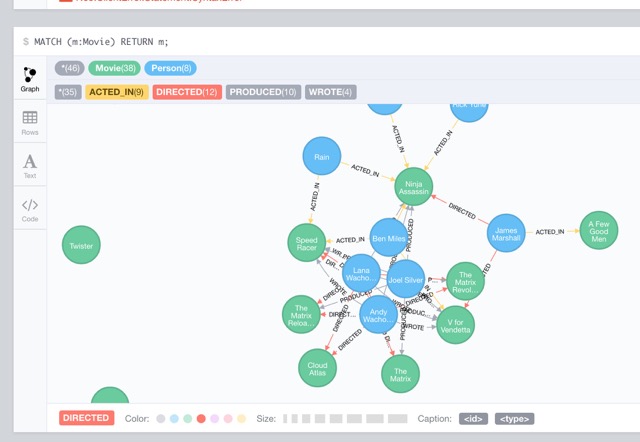
Data visualization tools like Neo4j can aid plans in data cleansing and planning regressions for machine learning techniques